Estimative language is a cornerstone of any good cyber threat intelligence report. It allows analysts to make clear, precise, and transparent assessments about the likelihood of an outcome or event so key stakeholders can make informed decisions. Without it, the lines between judgment and fact become blurred.
This guide will teach you what you need to start using estimative language in your threat intelligence reports and accurately assign a confidence level to your assessments. You will learn what estimative language is, its importance, and its three main components.
I will also share practical advice on using estimative language in the real world so you can begin implementing it today. Let’s jump straight in and start using estimative language!
What is Estimative Language?
Cyber threat intelligence (CTI) is all about making assessments. An assessment is a structured evaluation of the available information that produces a judgment or conclusion about a specific topic, event, or issue.
For example, “Based on the available intelligence, we conclude that this attack is linked to an ongoing phishing campaign targeting the financial sector known as Bob’s Big Phish.”
Do you see the problem with a statement like this?
If you’re a team leader or executive reading this assessment, you might be thinking:
- How confident are you that it is Bob’s Big Phish?
- Are you certain this phishing campaign is just targeting the financial sector?
- What is the likelihood that this is a new attack?
Questions like this are why estimative language (aka estimative probability) exists. Using estimative language, an analyst can add context to their intelligence assessments and unambiguously separate facts (evidence) from their own judgments.
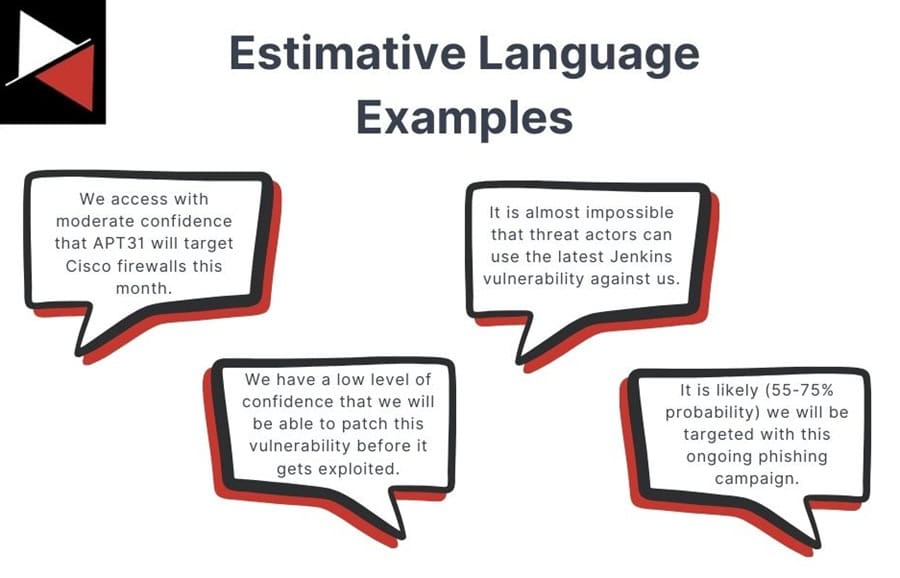
Estimative language consists of carefully chosen words that convey the confidence, certainty, or likelihood of an assessment’s conclusion. It allows a cyber threat intelligence analyst to express the reliability of their judgments based on the available evidence. This enables key decision-makers to better understand the context and reliability of the presented information.
High confidence assessment
Using the Analysis of Competing Hypotheses method, you determined that there is a lot of corroborating evidence that supports a single hypothesis and little for any others.
Moderate confidence assessment
There is a plausible and reasonable basis for a hypothesis to be true. However, your information is incomplete, or you are over-reliant on a single piece of evidence being true.
Low confidence assessment
You have a most likely hypothesis, but it is based on limited, fragmented, or uncorroborated information. A lack of evidence makes its reliability questionable at this time.
So, estimative language can help you express your confidence more clearly, but what are some other reasons to use it?
History lesson: Estimative language is based on a 1993 paper by the CIA titled Words of Estimative Probability. This whitepaper argues for “consistent, unambiguous usage of a few key odds expressions” to describe the reliability of intelligence. Since publication, these have become ubiquitous in the intelligence community and were later adopted by cyber.
Why is it Important to Use Estimative Language?
Estimative language is not just about expressing your confidence (even though this is a major advantage). It is also about being clearer, more precise, and transparent in your CTI reporting.
Clarity
Analysts can use estimative language to communicate their level of certainty in their assessments clearly. This minimizes potential misunderstandings when analysts need to interpret the available information and ensures intelligence consumers are given more context to inform their decisions.
Precision
Estimative language uses standardized terms, descriptions, and confidence levels so analysts can be precise in their communications. This helps key decision-makers better understand the intelligence they are given and allows intelligence to be shared with the wider community using a common language.
Transparency
Intelligence assessments should be transparent. They should be unambiguous, clearly report the facts, and show how analysts reached their conclusions. Estimative language helps analysts do this by adding nuance to the information provided.
“I have high confidence this was threat actor X because they used TTPs ABC, the traffic originated from country Y, and there are ten correlations with campaign Z.” The conclusion is that it is threat actor X and the facts are the TTPs, country, and correlations. This allows for a high-confidence assessment that separates facts from judgments.
In summary, here are the key benefits estimative language provides analysts and decision-makers.
- Clear communication: It allows analysts to clearly communicate their confidence in their assessments or the likelihood of an event occurring. This adds valuable context and implications key decision-makers need to be aware of.
- Promotes precision and transparency: Precise language helps accurately convey an analyst’s degree of certainty using supporting evidence that minimizes potential misunderstandings between facts and judgments.
- Aids decision-making: Estimative language provides nuanced insights that consider the reliability of information used to make intelligence assessments. This allows decision-makers to make better-informed decisions.
- Offers a common language: A common language for confidence levels in CTI reporting facilitates better communication among analysts, stakeholders, and organizations.
Let’s explore how to start using estimative language in your intelligence assessments.
The Key Components of Estimative Language
Estimative language can be used in various ways. It can describe the probability of an event or outcome, attach a confidence level to an assessment, or qualify an assessment with additional nuance.
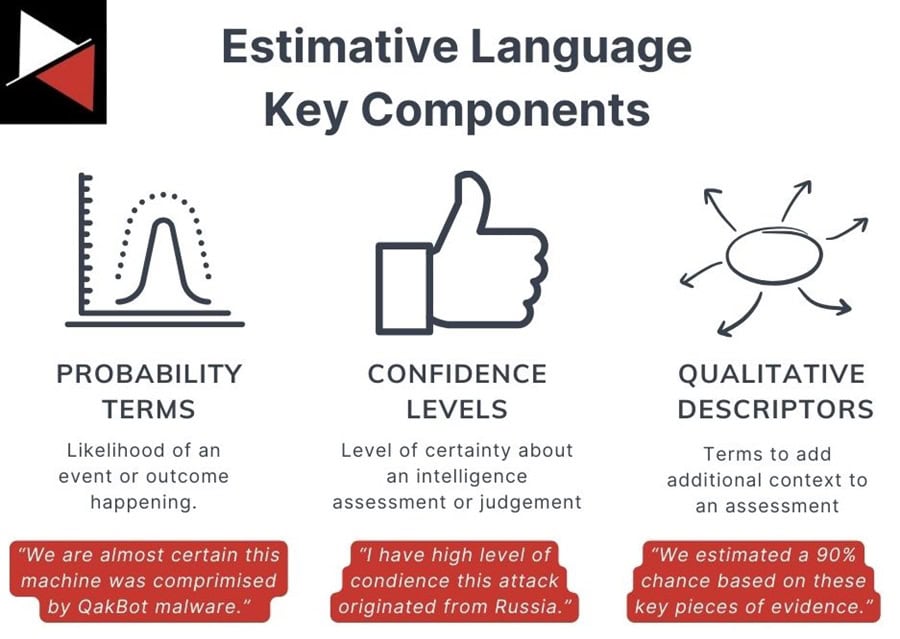
Probability Terms
When describing the likelihood of an event or outcome, you can use the following probability terms. These convey the degree of certainty you have about a specific prediction, allowing your intelligent consumers to weigh your assessment more accurately in their decisions.
Common probability terms include:
- Almost Certain: This event is very likely to occur (95-100% probability).
- Highly Likely: There is a strong probability this event will happen (75-95% probability).
- Likely: There is a greater than even chance this prediction will come true (55-75% probability).
- Possible: There is a roughly even chance of the event occurring (45-55% probability).
- Unlikely: There is a less than even chance the event will happen (25-45% probability).
- Highly Unlikely: A low probability of the prediction coming true (5-25% probability).
- Almost Impossible: Almost no chance of the event occurring (0-5% probability).
These are useful when you need to make a future prediction based on the current information available, be it evidence or trends. For instance, how likely is it you will see a new malware campaign exploiting vulnerability XYZ?
You can assess the probability of an event or outcome using statistics, past trends, or available evidence. Don’t get too caught up in putting a number on it. So long as you assign some objectivity to your judgments.
Confidence Levels
Confidence levels indicate the level of certainty you have about your assessment. They are more general than probability terms and fall into three main buckets:
- High Confidence: When an assessment is backed by high-quality information supported by many intelligence sources or multiple corroborating pieces of key evidence. This makes the assessment reliable and verifiable.
- Moderate Confidence: This is when the assessment is plausible and has a reasonable basis for being true. However, the information may not be complete or over-reliant on certain pieces of evidence. Analysts will make a moderate confidence assessment when they have supporting evidence for a hypothesis, but intelligence gaps prevent them from reaching a high confidence threshold.
- Low Confidence: When an assessment is speculative or based on limited, fragmented, and uncorroborated information despite it being the most likely hypothesis (using the Analysis of Competing Hypotheses). The lack of evidence makes its reliability questionable at this time.
An example of a high confidence assessment would be, “We assess with high confidence that the adversary will target critical infrastructure in the next six months, based on corroborated intelligence from multiple sources.”
If the sources were few or their reliability was questionable, you could scale this down to moderate confidence. Conversely, if there was only one source and they were unreliable, you could make a low confidence assessment.
You should use confidence levels in your assessments when evaluating multiple intelligence sources, data points, or pieces of evidence and judging the totality of the information you have been given.
Qualitative Descriptors
Qualitative descriptors are terms you add once you have used a probability term or confidence level to qualify why you gave that degree of certainty. For instance, if you say you have high confidence in your assessment, you will use a qualitative description to clarify why.
“I assess with a high level of confidence that we will be attacked by APT42 because… [qualitative descriptor] …”
Common qualitative descriptors include:
- Indicators and facts: These are key pieces of observable evidence that point towards a specific outcome (e.g., “We assess the threat actor has ties to Russia because the IP address came from Russia”). The more pieces of supporting evidence you can gather, the more confidence you can have in your assessment.
- Corroboration of evidence: Corroboration happens when new pieces of evidence support an existing hypothesis that is already backed by evidence. The more evidence you can gather that points to a single hypothesis, the more confidence you can have in your assessment.
- Statistical estimates: When you lack evidence to support a hypothesis, you can base your confidence (or probability) on a statistical estimate of an outcome being true. For instance, if a well-known vulnerability is being exploited in the wild and you know your systems are vulnerable, a threat actor will likely target these systems you own.
Adding a qualitative descriptor to your assessments helps the intelligence consumer understand how you reached the confidence level you did and how much weight your assessment holds. Without them, your assessment is just an opinion.
Practical Advice on How to Use Estimative Language
If you are new to estimative language, understanding when, what terms to use, and how to qualify your confidence using descriptors can be challenging. To help you get started, here are some practical tips.
Understand the Standard Descriptors
Familiarize yourself with the key probability terms, confidence levels, and qualitative descriptors used to describe how certain you are about an assessment.
- Probability terms = almost certain, highly likely, possible, unlikely, highly unlikely, and almost impossible.
- Confidence levels = high, moderate, and low.
- Qualitative descriptors = indicators and facts, corroboration of evidence, and statistical estimates.
Choose the Type of Descriptor
Choose the estimative language you use based on the assessment you are making. If you are assessing the likelihood of an event occurring, use a probability term. If you are evaluating the quality and reliability of the information supporting your assessment, use a level of confidence.
“It is highly likely that the software vulnerability will be exploited within the next month” (probability) vs. “We have moderate confidence in this assessment due to limited corroborating sources” (confidence).
Provide Context and Justification
Explain how you reached a probability or confidence level using a qualitative descriptor. This descriptor can provide context (e.g., based on THIS piece of evidence) or justification (e.g., based on X amount of corroborating evidence). You can use facts, corroborating evidence, or statistical estimates to explain how you came to your conclusions.
Be Clear and Concise
Separate the facts and evidence in your assessments from your judgments and conclusions. You want to avoid ambiguous terms and ensure your report is straightforward and easy to understand.
“I have come to this conclusion [state conclusion]. I have a [high/moderate/low] confidence in this conclusion because of these facts [state facts].”
Tailor the Language to the Audience
Not all intelligence consumers are the same. You may provide an intelligence assessment to a security operations team that needs operational and tactical intelligence. On the other hand, you could be providing an assessment to an executive about whether a new policy will affect the company’s marketing strategy.
You must tailor the language you use in your assessment to the audience consuming it. This is fundamental to disseminating threat intelligence and ensuring decision-makers find it valuable. In fact, there are entire tools built to help you better structure your reports based on the target audience (e.g. MITRE’s CTI Blueprints project).
You can learn more about the different types of cyber threat intelligence in What is Cyber Threat Intelligence? A Quick Guide.
Review, Revise, and Educate
Estimative language should be fluid and change as new information becomes available. If you can scale your moderate confidence assessment to a high level one because new evidence comes in, you should (and you should let others know about it).
Your intelligence assessments should reflect the most recent intelligence. As new data is added to your report, the language used to describe the likelihood of an outcome should also be revised to reflect these changes.
It is also important to train employees in using estimative and key decision makers on what estimative language means in the reports you provide them with. You could do this through shared documentation detailing the probability terms and confidence levels or training programs focusing on report writing.
Conclusion
Estimative language is a fundamental tool in your CTI reporting toolbox. It allows you to add clarity, precision, and transparency to your intelligence assessments so key stakeholders know the likelihood of an outcome or event occurring. The extra context provided by adding estimative language can be the difference between a well-informed decision and falling down a rabbit hole.
This guide showcased estimative language, its importance, and how to use it with probability terms, confidence levels, and qualitative descriptors. It even included practical advice on using estimative language in the real world so you can start using it in your reports today.
Enjoy using this new knowledge and improving your reporting skills!
Frequently Asked Questions
What is the Purpose of Estimative Language?
Intelligence assessments include estimative language to add a degree of certainty to a report. This could be how certain an analyst is that an event or outcome will happen or how certain they are of their judgment about an event based on the information available.
It is a critical part of any cyber threat intelligence report. It allows analysts to provide clear, precise, and transparent assessments that separate facts from judgments so key decision-makers can take the appropriate action.
What Percentage is Highly Likely?
The probability term “highly likely” describes an event or outcome with a strong probability of occurring (75-85% probability). This is based on the information available to the analyst at the time or using a statistical estimate.
What Percentage is Considered Unlikely?
The probability term “unlikely” describes an event or outcome with a less than even chance of happening (25-45% probability). This is based on the information available to the analyst at the time or using a statistical estimate. An unlikely event may become “possible” or “likely” as new evidence becomes available.
Why is Accuracy Important in Intelligence?
Accuracy is one of the fundamental pillars of good threat intelligence, along with relevancy, timeliness, and the ability to take action. The reliability and consistency of the intelligence sources or evidence an intelligence assessment is based on will determine its accuracy. Analysts will use estimative language when describing this information to add context to their assessments and ensure the intelligence consumer can make an informed decision.